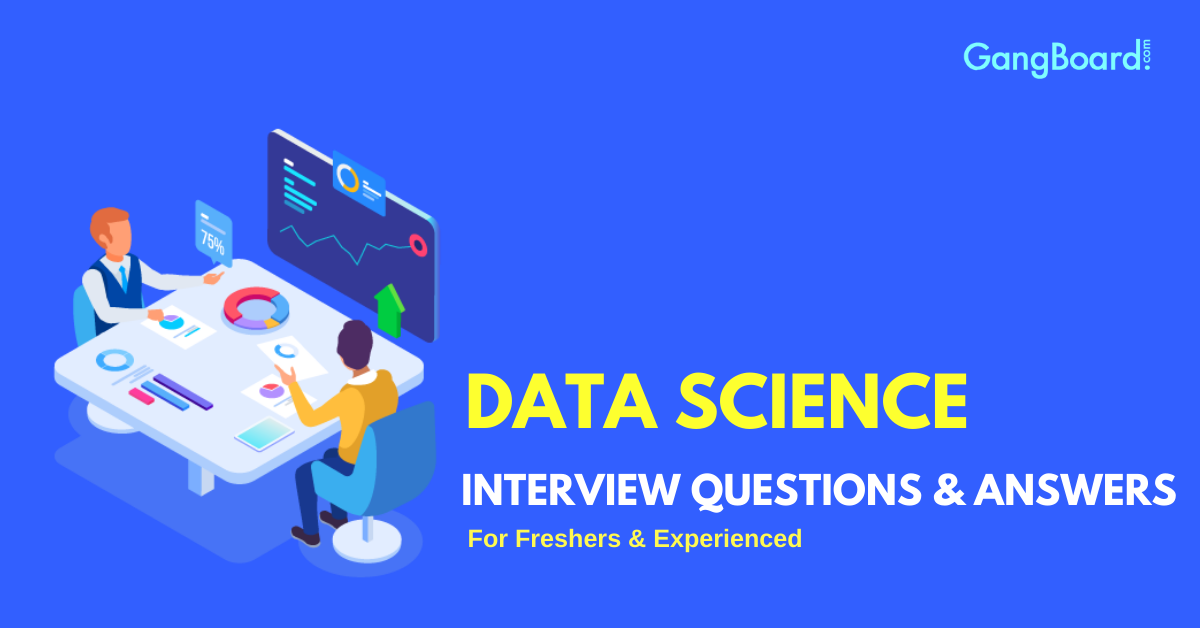
Data Science with R Interview Questions and Answers
Data Science with R Interview Questions and Answers
In case you’re searching for Data Science with R Interview Questions and answers for Experienced or Freshers, you are at the correct place. There is a parcel of chances from many presumed organizations on the planet. The Data Science with R advertise is relied upon to develop to more than $5 billion by 2020, from just $180 million, as per Data Science with R industry gauges. In this way, despite everything you have the chance to push forward in your vocation in Data Science with R Development. GangBoard offers Advanced Data Science with R Interview Questions and answers that assist you in splitting your Data Science with R interview and procure dream vocation as Data Science with R Developer.
Best Data Science with R Interview Questions and Answers
Do you believe that you have the right stuff to be a section in the advancement of future Data Science with R, the GangBoard is here to control you to sustain your vocation. Various fortune 1000 organizations around the world are utilizing the innovation of Data Science with R to meet the necessities of their customers. Data Science with R is being utilized as a part of numerous businesses. To have a great development in Data Science with R work, our page furnishes you with nitty-gritty data as Data Science with R prospective employee meeting questions and answers. Data Science with R Interview Questions and answers are prepared by 10+ years of experienced industry experts. Data Science with R Interview Questions and answers are very useful to the Fresher or Experienced person who is looking for a new challenging job from the reputed company. Our Data Science with R Questions and answers are very simple and have more examples for your better understanding.
By this Data Science with R Interview Questions and answers, many students are got placed in many reputed companies with high package salary. So utilize our Data Science with R Interview Questions and answers to grow in your career.
Q1) Explain Data Science? uses
Answer: Data Science is the combination of the various scientific method, processes knowledge like statics, regression, mathematics, computer science, algorithm, data structure, etc. With the help of data science, we can get knowledge about various technologies like data mining, storing, purging, archival, transformation.
Use: It is used to modify the data of various types like structured, unstructured, semi-structured for getting details.
Q2) Define Bucket testing in Data Science?
Answer: It is considering as A/B testing in Data science. It is used in application to compare and test the two versions for checking the performance of the version. A/b testing is used to imagine the outcomes.
Q3) What is used for the multilayer neural network?
Answer: Backpropagation is used for the error to move from the end of the network to all weighs. It is used to modify the according to the previous function.
Q4) What is Recurrent neural network?
Answer: A Boltzmann machine used to solve the opposite problem in computer., It can show the difficulties in the training data. It is used to improve the weights and solve the problems. This learning algorithm becomes faster by learning one layer of feature detectors at a time.
Q5) How to convert inputs into outputs?
Answer: By Autoencoders with fewer errors to keep output and input very close. A deep neural network for producing the coating of input and output. It is divided into two parts encoder and decoder.
Q6) How to introduce non-linearity into the neural network?
Answer: The Activation function gives an output based on inputs. It controls the activation of a neuron. To introduce non- linearity into the output of a neuron is the motive of the Activation function.
Q7) Describe Supervised learning?
Answer: Supervised learning is used to map the labels of input and output, regression. Data scientist performs to teach the algorithm for the conclusion. It is used to teach the algorithm which is labeled with the correct Answer.
Q8) Explain Unsupervised learning?
Answer: – Unsupervised learning is known for clustering, estimation of density and representation learning. We cannot compare the model performance in unsupervised learning methods. It is used for analyzing exploratory and reduce the dimension.
Q9) What is a computational model?
Answer: An artificial neuron network is a nonlinear statical data to explain the difficulties between input and output. The model is based on the functions and the structure of a biological neural network. The changes are made on input and output, it is forced by the movement of information.
Q10) Name the variants of Backpropagation?
Answer:
- Mini- batch Gradient Descent – It is for optimizing the algorithm.
- Stochastic Gradient Descent Single training is used to calcite gradient and the updating of parameters.
- Batch Gradient Descent The gradient is calculated for the whole dataset and to achieve the updating of each iteration.
Q11) What are the set of algorithms?
Answer: Artificial Neural networks contain a revolutionary machine learning and are inspired by biological neural networks. The neural network modifies the changes to gain suitable results by the network without repeating the output rules.
Q12) Name the error decided by the researcher?
Answer: Selection bias is the error where the selection of participants is not random. It is known as the deformation of statistical analysis. The conclusion of the study is not accurate when the selection bias is not an account.
Q13) Tell the types of Bias in Data science?
Answer:
- Confirmation Bias
- Rescue Bias
- Orientation Bias – It returns the situation of recording and experimental error to hold up the hypothesis.
- Cognitive Bias – To decide on the pre-existing factors.
- Selection Bias – To change the choice of data sources on the pre-existing factors.
- Sampling Bias – Cause by non-random sample of the population.
- Modeling Bias – For changing the Data science models by the set of biased for the difficulties. We can choose the wrong data, variables, algorithms, metrics.
Q14) How to avoid Bias in data science?
Answer:
- Trace all data sources and profiles
- Check the data having qualitative information.
- Review the data transformation and effects on the populations.
- Trace the development of the data understanding and work products.
Q15) Define logistic regression?
Answer: logistic regression is a statically analyzer for forecasting the result of the dependent variable. In machine learning, logistic regression has many applications. And algorithm helps to know the winning candidate in elections.
Q16) Explain the Recommender Systems?
Answer: Recommender Systems is the way to connect the user and content from each other. With the help of Recommender Systems, the user get the most suitable information about the product. It is mostly used in movies, blog posts, communities.
Q17) What is the role of Cleaning Data?
Answer:
- It helps to raise the correctness of the model in machine learning
- We can clean the data from multiple sources to convert its format so that data scientists can work easily.
- It is a cumbersome process, as the data source rises the time increases for cleaning.
- To clean the data, it needs 80% time.
Q18) Explain Normal Distribution? It’s characteristics
Answer: It is the grouping of data sets. The data values collection in the middle of the range. Blood pressure Intelligence, height automatic follows the normal Distribution
- Unimodal
- Symmetric
- Asymptotic
- Mean, median, mode
Q19) Define Linear Regression?
Answer: – Linear Regression specifies the link between one or more forecasting and one outcome variable. It is used to guess, analyze and model. It is the way to imagine the score of a variable y and X.
Q20) How to validate the accuracy of a classifier?
Answer: Sensitivity is for validation of logistic, SVM, Random, Forest. It is called Predicted True events/Total events. The events are true and model. And the calculation is uncomplicated.
Q21) What is overfitting?
Answer: – Random error is defined by overfitting. Overfitting takes place when a model is difficult like numerous parameters are related to many observations. An overfit model has a low predictive performance.
Q22) What is underfitting?
Answer: When an underlying trend of the data is not captured by a machine learning algorithm of the statistical model. It also has very low predictive performance. And it occurs when a linear model is fitted to non-linear data.
Q23) How to target the population spread in a wide range?
Answer: By using Cluster sampling, it is known as a possibility sample for each sampling unit. This is used to divide the population into groups. It the group of elements used for market research.
Q24) What is the experimental design Technique?
Answer: A power analysis is known as the technique of experimental design. It is used to regulate the influence of a sample size.
Q25) What is the work of Database Design?
Answer: – It is used for creating output for the detailed data model. Database Design contains the complete logical models, physical deigns and the storage parameters.
Q26) Who creates the conceptual model?
Answer: Data modeling, it is the initial step for designing a database. The model is connected to different data models. It helps in operating the conceptual stage to the logical then to the physical schema with the systematic method
Q27) Explain the Random forest model?
Answer: To merge the numerous models for getting the final output. The multiple decision trees are joined together. And the trees are creating blocks of random forest model.
Q28) What is known as the part of the training set?
Answer: A validation set is considering a training set. The selection of the parameters like weighs is done by the validation set. And used to keep away from the overfitting of the model is created.
Q29) What is Test set?
Answer: – The performance of a trained machine learning model is to check and judge by the Test set. It also analyzes the guessed power and generalization. A test set is curated contains sampled data of different classes.
Q30) What is the goal of cross-validation?
Answer: It is a way to check the outcomes of statistical analysis for creating an independent dataset. It is used in the background where we predict the objectives. To evaluate the accuracy of a model achieving the practice.
Q31) Define Collaborative filtering?
Answer: For creating the private recommendation on web collaborating filtering is used. It is the process to filter the recommender systems for searching patterns and information by interacting with numerous agents, data sources and viewpoints.
Q32) Mention the steps in an analytics project?
Answer:
- To understand the difficulties of the business.
- Search the data which is close to it.
- Justify the model by the use of the new data set.
- Trace the results for examining the performance of the model which is up to time.
- Start running the model after constructing the data.
Q33) Why we do Resampling?
Answer: –
- To rate the accuracy of the sample statistics.
- At the time for performing significance tests then substituting labels on data points.
- Random subsets such as bootstrapping and cross-validation are used to prove models
Q34) What is the time of Algorithm updating?
Answer:
- When you need to develop data streams by the base
- At the period of modification of the underlying data source.
- When the case of non-stationarity occurs.
Q35) Define the Star schema?
Answer: It is a common database schema having a central table. In this, the one fact table hints the numerous dimension tables, when it is considered as the diagram, star. Used widely among the data warehousing schemas.
Q36) Explain the Law of large numbers?
Answer: in the theory of statistics and probability is to define the results for redo a similar experiment many times. When the same experiment is replay separately a large number of theorem states. As the trails expand the results come closer to the expected value.
Q37) Tell about Confounding Variables?
Answer: In the statistical model they are considered as extra variables. It is related straight or again for the dependent and independent variables. The evaluation does not account for the confounding factor.
Q38) What is used to analyze industrial accidents?
Answer: By the use of Root cause analysis, It is not only to check the difficulties in an industrial area but also in another field. It is also known as a problem-solving technique for difficulties or faults.
Q39) Define probability in Data science?
Answer: It provides an opportunity to happen something and to calculate as for the event We use this regularly without consuming speaks and applying the chances of the work.
Q40) Define Statistics in Data Science?
Answer: Every entrepreneur needs a strong grip of Statistics. It is the research of gathering, explanations to examine the management and the data of a particular organization, We can say it used for the growth of the business and to resolve all the difficulties.
Q41) What is used to decide the statistical? It’s types
Answer: Hypothesis testing is the way for the statistical decision but the use of experimental data. It is like an assumption for the parameter of the population.
- Null and Alternative Hypothesis
Q42) What is important for statistical analysis?
Answer: Interpolation and Extrapolation are very essential for analyzing the statics.
- Interpolation To consider the value or the set of values in the context is known as Interpolation. We can fill the missing data like upscaling images or creating statistical models with the help of interpolation. It is the straight line between two or more points.
- Extrapolation It controls the definite value between the confirmed set or sequence of values. It is the way to conclude anything by the available data.
Q43) What is an n-dimensional vector?
Answer: A feature vector is a numerical feature to show some object. Feature vectors are for serving numeric or symbolic characteristics. It is very easy to consider an item in mathematics.
Q44) How to maintain the unstructured data?
Answer:- Hadoop gives the capacity to handle the huge unstructured data. The different new extension of Hadoop like Mahout and PIG gives features to examine and implementing the machine learning algorithms on the vast data. So that data scientists can easily handle all the forms of data.
Q45) How to reduce the error by fitting a function?
Answer: By regularizations can decrease the error by fitting a function on the training set. It is for removing overfitting. At the time of training, there is the possibility of model learning noise or the data points without showing any property of true data. This is considering as overfitting.
Q46) How to maintain the hardness or softness of large margin classification?
Answer: By using Cost Parameters we can handle our large margin classification. It decides the performance of the data. Smooth decision surface is used at a low cost. The higher cost is used for arranging more points.
Q47) What is the use of Term Frequency?
Answer: To recover and mining information. It is like a weighing factor for searching the fundamentals of the word to a document. It grows in number as the word occurs.
Q48) What is a rectangular distribution?
Answer: A uniform distribution contains continues chances. It has two parameters A is minimum and b is maximum. It is used to decrease random variables.
Q49) Name the carrier and jobs area of data science?
Answer:
Carrier
- Econometrician
- Biostatistician
- Mathematician
- Consultant
- Professor
- Statistics trainer
- Content Analyst
- Data Analyst
- Risk Analyst
- Business Analyst
Jobs area
- Ecology
- Election
- Economics
- Census
- Crime
- Film
- Sports
- Tourism
Q50) Define Unequal Data?
Answer: The group of balanced data for classification difficulties is known as special classes. The class distribution is between the classes is defined as two types
- The majority class is negative
- The minority class is positive
It is a new issue for this type of set data. A consistent training package is examined by the worthy classification protocols.