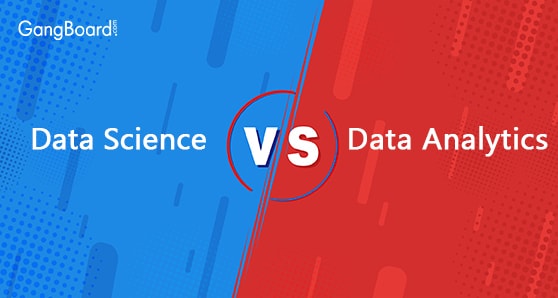
Data Science and Data Analytics
Difference Between Data Science & Data Analytics
A Tech aspirant who is confused between Data Science and Data Analytics? Not a problem! This common dilemma occurs due to the domain relevance. However, as similar as they sound, they are actually quite different in reality.
If you are someone who works in an IT firm, you might hear these terms quite often. With the emergence of Big Data, Data Science and Data Analytics have made an inseparable place in growing IT companies.
The demand for Data Analysts and Data Scientists is inevitable considering the fact that growing tech firms cannot make the mistake of ignoring them if they need to surge in the competitive IT sector.
Let us explore the meaning and significance of these terms and what makes them different from each other.
What is Data Science?
Data Science comprises of various processes, tools, and algorithms to exploit structured or unstructured data in order extract useful knowledge. Here, it is important to understand that Data Science is greater than a simple software, visualization method, or statistics, which is a common misconception.
The life cycle of Data Science begins with data discovery, which is to identify the resources and intent of the whole process. A data scientist needs to ask a lot of questions in order to understand the business needs of their clients.
After that comes the data acquisition process where data is acquired from heterogeneous resources such as logs, database, servers, and more. Once the data is acquired a data scientist needs to clean and transform this data to make it usable as raw data may contain missing fields, misspelled attributes, duplicates, human errors, etc.
Another thing to keep in mind is that all this acquired data is collected from disconnected sources, which makes it even more difficult to unite and work with. However, a data scientist examines this data and comes up with better models in terms of both analytics and machine learning. Moreover, this process helps a business to identify hidden patterns lying underneath the unstructured data and find suitable models.
Overall, the whole process is quite exhausting keeping the several stages in mind. Let us see a real life example to easily understand what Data Science is.
Data Science – A Real-life Example
Majority of tech, digital marketing, and advertising companies use data science algorithms to target specific advertisements to specific audience. A more appropriate example would be online stores such as Amazon, Flipkart, Snapdeal, which use their large volume of data to predict the likely purchases of a consumer on the basis of their search, cart, wishlist etc.
What is Data Analytics?
Data Analytics refers to the statistical analysis of data to come up with business-essential information and solve problems. The process may comprise of cleaning, transformation, and modeling of data.
The life cycle of Data Analytics is quite simpler and shorter compared to that of Data Science. It begins with acquiring and processing data, then summarizing that data for insights, and lastly, building visual insights and reports.
There are mainly three types of Data Analytics
- Descriptive
- Predictive
- Prescriptive
Descriptive Analytics
It refers to building models that provide an insight in current and historical data and show the performance of the company in various areas such as finance, inventory, customers etc. However, it does not give insights regarding future, which brings us to the second type – Predictive Analytics.
Predictive Analytics
It is used to make informed decisions utilizing historical data such as which type of customers to target or which product can be more profitable.
Prescriptive Analytics
It is about providing advice and knowledge-based proposals to make better decisions on the possible outcomes and optimizing production, inventory, marketing approach, etc.
Data Analytics – A Real-life Example
Data Analytics is applied more in IT, food, healthcare, travel and similar other industries. Any fast food chain uses data analytics to understand the demand of the product according to time and preferences. In fact, even the menus are decided according to data analytics!
i.e. people would rather just have a single cheap burger than buy burger, fries, and coke individually. However, understanding the constant preference of those three, a combo is the result of data analytics, which saves a couple bucks of the customers (hardly enough to make your pocket happy but is mentally satisfactory) and keeps the sale high.
Data Science vs Data Analytics
To begin with, Data Science is a vast term comprising of multidisciplinary names such as machine learning, business analytics, software engineering, data analytics and more, which makes it an umbrella term that also involves data analytics.
Data Scientist vs Data Analyst – Job Profile
The difference between the job profiles of a data scientist and data analyst is quite distinguishable. While a data scientist uses data to forecast the future and identify hidden patterns, a data analyst comes up with meaningful insights by statistically analyzing data, often in a visual form.
Who Do They Work For?
A data scientist, besides the term scientist, does not work for academia nor does he publish research papers. In fact, data scientists can be found in divergent industries such as for tech giants like Google, Yahoo, MSN, where they continually work on their search algorithms to come up with more intent-based results. Moreover, financial services like banking, mutual funds, insurance also need data scientists to discover patterns that are more profitable.
Furthermore, all e-commerce websites, whether they are giants like Amazon or even a small-scale one, need to incorporate data science techniques to stay alive in the competitive market.
Moving on to the data analyst, a data analyst works foremostly in the IT industry to analyze the data and subsequently build meaningful insights. Data analysts are also required in domains such as healthcare and travel industries to deal with executional challenges.
The Difference in Skill Set
Both data scientists and data analysts require some common skills to deal with the vast amount of data. Programming languages such as Python, SAS, or R are essential and so are the mathematical and statistical skills to analyze the data.
However, a data scientist also requires storytelling skills to make complex information easier for a non-technical person to understand. On the other hand, a data analyst should have data wrangling skills, which is not vital for a data scientist to survive.
Dealing with Big Data, a data scientist also requires a decent understanding of Hadoop and SQL, which is not necessary for a data analyst. In the same manner, data scientists working in AI firms need to have a profound knowledge of machine learning and relevant algorithms.
How Much Do They Earn?
The salary difference between a data scientist and data analyst is drastic. Looking at the median, a data scientist earns almost double than a data analyst, which is mostly due to the complexity of the work.
While a data scientist in the US earns about $127,774 per year, a data analyst only earns $68,501 on an average, according to a recent estimation by Indeed.
Though the salary difference may make an aspirant to incline for Data Science, it is important to understand that both the fields offer amazing opportunities to those who comprehend the nitty-gritty of the work. Moreover, the median salary just indicates the earnings of an average employee, the upper boundaries of wages are much higher than the average pay. Frankly, a data analyst could be earning even more than a data scientist and vice versa.
Therefore, at GangBoard, we suggest you figure out your skill set and choose the field that is more suitable for you if you wish to succeed in your further endeavors.
We hope that this article was helpful for you to understand the basic contrast between Data Science and Data Analytics. Let us know if you have any query regarding this article, we will be glad to satisfy your curiosity.