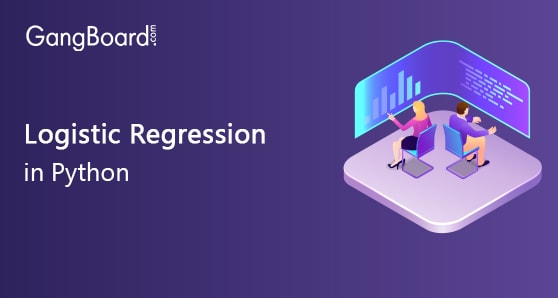
Logistic Regression
Logistic Regression
Scientifically speaking, Logistic Regression is the regression analysis to be conducted when we are faced with a binary or dichotomous dependent variable. It is a statistical tool developed by David Cox, a statistician, in 1958 which has been applied in Life Sciences since its early days and then extended to Social Sciences. Now it is being extensively used in the IT and Information domains.
What is Logistic Regression?
If you want to understand the term ‘Logistic Regression’, then you must understand it in simple mathematical terms: a binary Logistic model involves a dependent variable where only two values are possible; YES or NO, PASS or FAIL, COMPLIES or DOES NOT COMPLY, etc.
Logistic Regression derives its name from Logistic Function which is a sigmoid function that was employed to study population growth in ecology by the statisticians. It has an S-shaped curved and can convert any number within the limits of 0 and 1.
The need for Logistic regression arose because Linear regression was unable to cater to problems involving classification, for example: Whether an email is a spam or not?
The problem arises because Linear regression is unbounded while Logistic regression values strictly follow the range of 0 or 1. While Logistic regression uses an equation similar to linear regression, the primary difference is that the modeled output is binary (0 or 1).
Logistic Regression Classification
A classification of Logistic Regression based on the category of the target variable is as follows:
Binomial:
Where the variable can only have 2 possible values namely, 0 or 1, which represent outcomes such as “yes or no”, “pass or fail”, “alive or dead”, etc.
Multinomial:
The variable can have 3 or more different possibilities that aren’t ordered. Examples: “Plant, Animal, or Human”, “Reptile, Amphibian, or Fish”.
Ordinal:
The variable has ordered categories ranked according to magnitude, such as, “Worst, Bad, Average, Good, Very Good, Excellent”. The categories are designated as 0, 1, 2, 3, 4, 5, etc.
Estimation Technique
The most frequently applied Binary logistic regression employs Maximum Likelihood Estimation (MLE) for its estimation. This is in contrast to linear regression which makes use of the Ordinary Least Squares (OLS) method.
The latter is completely iterative, which means that it begins by guessing the best weight for a predictor variable – the coefficient in the model.
It subsequently operates by the repeated adjustment in the coefficients to a point when there remains no room for additional improvement in the predictive ability of the outcome variable (0 or 1).
The Standard Logistic Regression is a sigmoid function with an ‘S’ shaped curve between the following limits:
As S approaches -∞ the value is 0
As S approaches +∞ the value is 1
The Logistic Regression Function Can be explained by the equation:
f(x) = L/(1+e ^-k(x-x0))
Where the various components of the equation can be denoted as,
L – Maximum value of the Sigmoid Curve
K – The sigmoid curve’s steepness
X0 – The x value of the curve’s mid-point
To obtain a standard logistic function we will need to plug in the values k=1,x0=0, L=1 and then we obtain the following equation:
S(x) = 1/1+ (e ^ – x)
This is the equation of the ‘S’ shaped sigmoid curve.
Logistic Regression for Machine Learning and Predictive Modelling
One of the many techniques that Machine Learning has adopted from Statistics is Logistic Regression to predict the dependent variable’s probability. Here, the dependent variable needs to be strictly binary in which the data is coded either as 1 (yes/ pass) or 0 (no/ fail). It allows the user to predict the increase or decrease in the probability of the given outcome, by a definite percentage, based on the presence or absence of a risk factor.
The following assumptions are made while dealing with Logistic regression:
- The dependent variable must be binary in the logistic regression of the binary type.
- The model must not be multicollinear, meaning that the variables must be independent of one another.
- Only significant/ meaningful variables are to be included.
- The operation is done for a large sample size.
Logistic regression’s applicability towards predictive analysis
If a student data is provided on hours of study and hours at play and a predictive analysis needs to be given such that a decision of pass or fail (1/ 0) is to be reached, linear regression won’t be of much help. Here we have 2 features- study hours and play hours and two result classes- Pass(1) and Fail (0).
It can be plotted on a scatter plot and no decision will be reached, but in order to reach the correct conclusion, a sigmoid function, the binary Logistic Function will need to be employed.
Machine Learning employs the same Sigmoid curve for predictive analysis, such that values are mapped on to probabilities. This function results in mapping any kind of real values into binary values between 0 and 1.
Selecting the Threshold:
After the values have been mapped into binary functions namely 0 or 1, the next task is to map it into the desired result (true/ false), (up/ down), etc. For that, we will need to set up a threshold value. The threshold ensures that all values above the threshold are mapped into 1 and all values below the threshold are inferred as 0.
The corresponding values are then the predictive outcome of the entire operation. As an example, if we have set a Threshold as 0.6 and obtained a value of 0.8, it will give the result as “Pass”, while if we obtain a value of 0.4, we will get the end prediction as “Fail”.
While making predictions, the confidence of the predictive element becomes stronger as the probabilities map out closer to a particular outcome.
For example, if the value is 0.9, the prediction of “Pass” is far stronger than say, if the probability is around “0.7”. Similarly, if the probability is around 0.1, the predictive outcome “Fail” is much stronger than if the probability is around “0.4”.
Application of predictive analysis
The applications of this advanced predictive tool have been seen worldwide and in various domains from Healthcare to Social sciences. However, the current use of Logistic Regression is highly focused on Machine Learning Algorithms for advanced analytics and research.